"Often I compare my own Fermi estimates with those of other people, and that’s sort of cool, but what’s way more interesting is when they share what variables and models they used to get to the estimate."
– Oliver Habryka, at a model building workshop at FHI in 2016
One question that people in the AI x-risk community often ask is
"By what year do you assign a 50% probability of human-level AGI?"
We go back and forth with statements like "Well, I think you're not updating enough on AlphaGo Zero." "But did you know that person X has 50% in 30 years? You should weigh that heavily in your calculations."
However, 'timelines' is not the interesting question. The interesting parts are in the causal models behind the estimates. Some possibilities:
- Do you have a story about how the brain in fact implements back-propagation, and thus whether current ML techniques have all the key insights?
- Do you have a story about the reference class of human brains and monkey brains and evolution, that gives a forecast for how hard intelligence is and as such whether it’s achievable this century?
- Do you have a story about the amount of resources flowing into the problem, that uses factors like 'Number of PhDs in ML handed out each year' and 'Amount of GPU available to the average PhD'?
Timelines is an area where many people discuss one variable all the time, where in fact the interesting disagreement is much deeper. Regardless of whether our 50% dates are close, when you and I have different models we will often recommend contradictory strategies for reducing x-risk.
For example, Eliezer Yudkowsky, Robin Hanson, and Nick Bostrom all have different timelines, but their models tell such different stories about what’s happening in the world that focusing on timelines instead of the broad differences in their overall pictures is a red herring.
(If in fact two very different models converge in many places, this is indeed evidence of them both capturing the same thing - and the more different the two models are, the more likely this factor is ‘truth’. But if two models significantly disagree on strategy and outcome yet hit the same 50% confidence date, and we should not count this as agreement.)
Let me sketch a general model of communication.
A Sketch
Step 1: You each have a different model that predicts a different probability for a certain event.
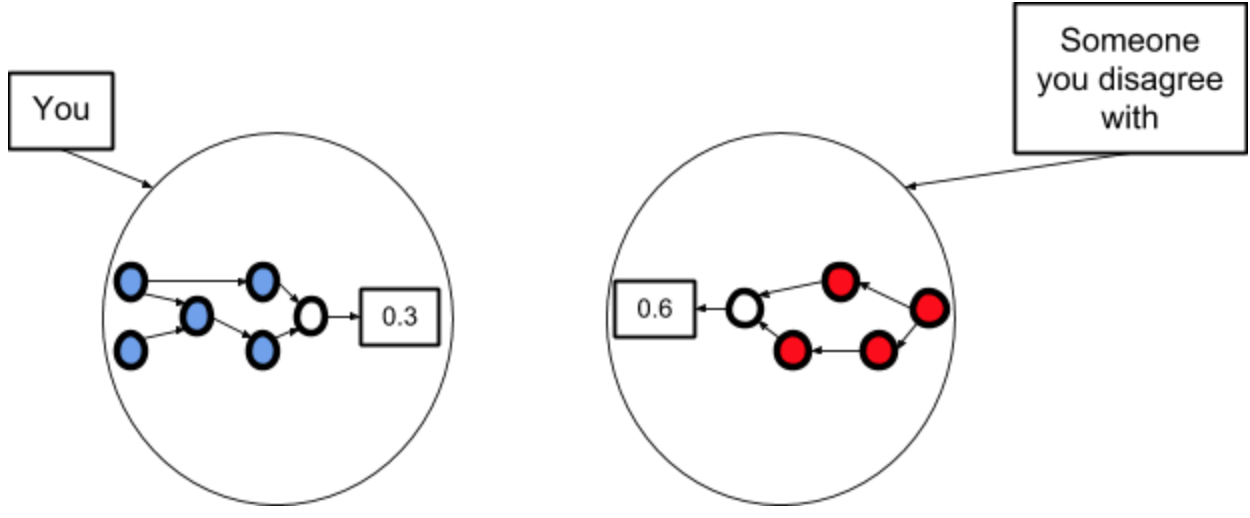
Step 2: You talk until you have understood how they see the world.
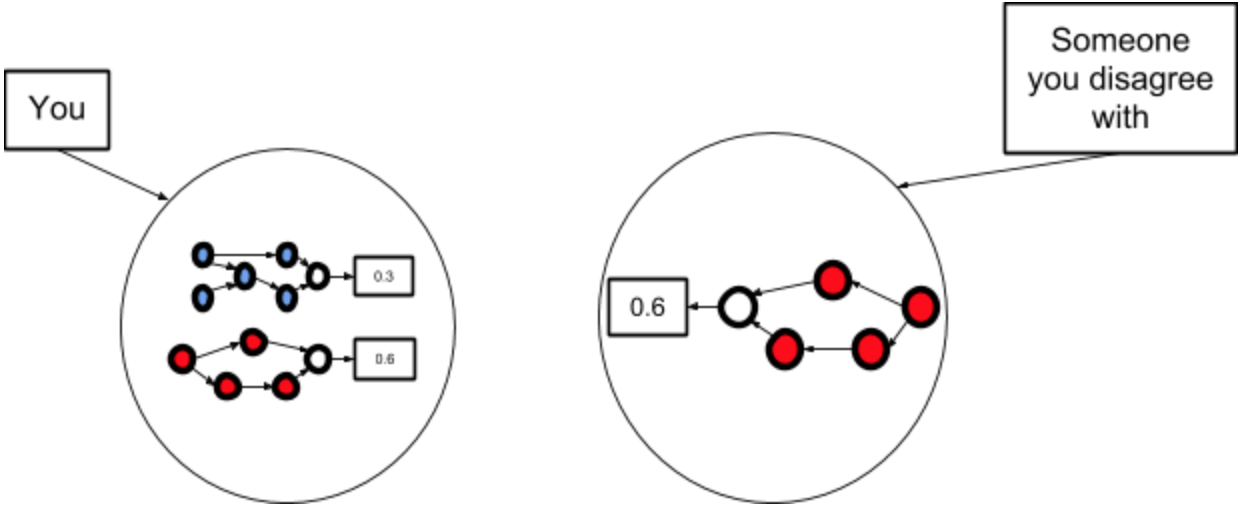
Step 3: You do some cognitive work to integrate the evidences and ontologies of you and them, and this implies a new probability.
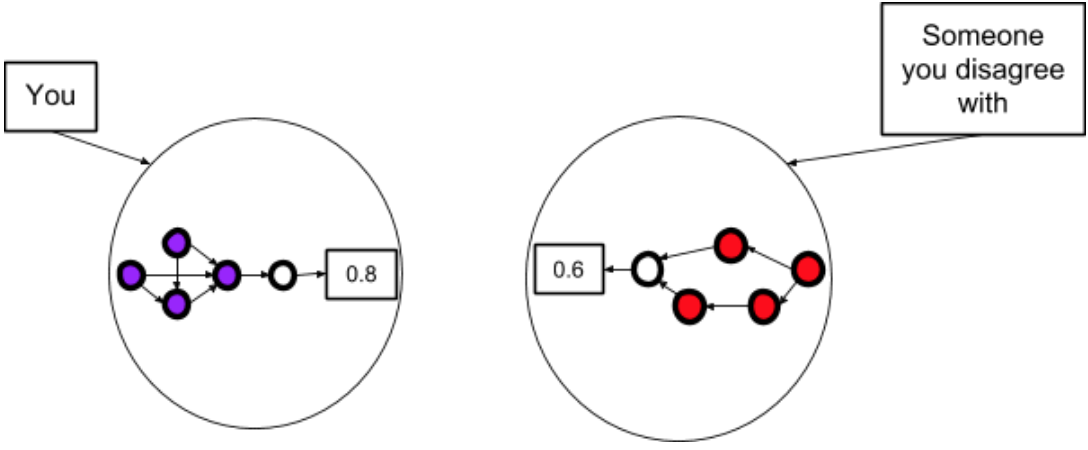
"If we were simply increasing the absolute number of average researchers in the field, then I’d still expect AGI much slower than you, but if now we factor in the very peak researchers having big jumps of insight (for the rest of the field to capitalise on), then I think I actually have shorter timelines than you.”
One of the common issues I see with disagreements in general is people jumping prematurely to the third diagram before spending time getting to the second one. It’s as though if you both agree on the decision node, then you must surely agree on all the other nodes.
I prefer to spend an hour or two sharing models, before trying to change either of our minds. It otherwise creates false consensus, rather than successful communication. Going directly to Step 3 can be the right call when you’re on a logistics team and need to make a decision quickly, but is quite inappropriate for research, and in my experience the most important communication challenges are around deep intuitions.
Don't practice coming to agreement; practice exchanging models.
Something other than Good Reasoning
Here’s an alternative thing you might do after Step 1. This is where you haven't changed your model, but decide to agree with the other person anyway.
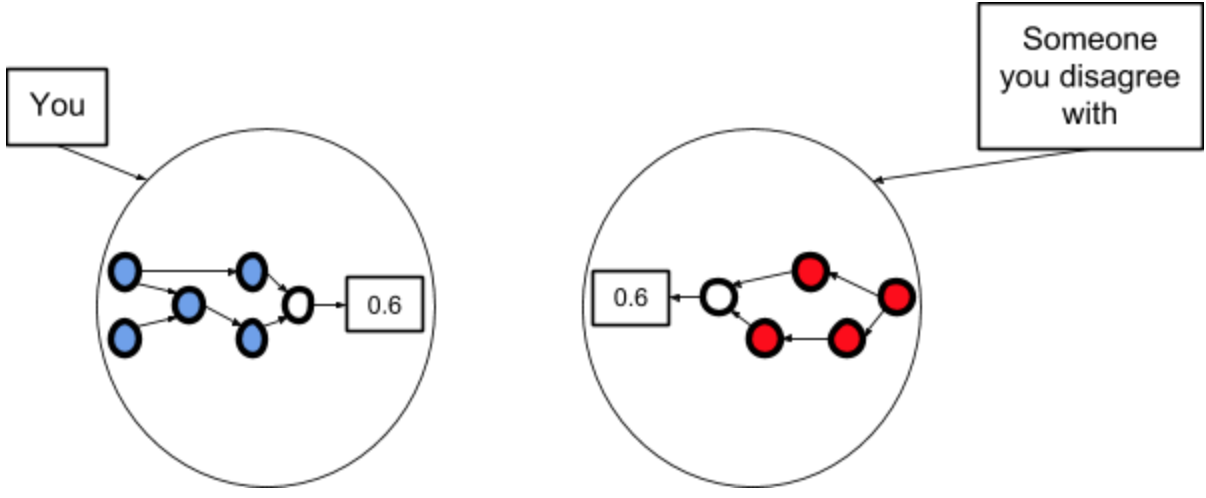
This doesn’t make any sense but people try it anyway, especially when they’re talking to high status people and/or experts. “Oh, okay, I’ll try hard to believe what the expert said, so I look like I know what I’m talking about.”
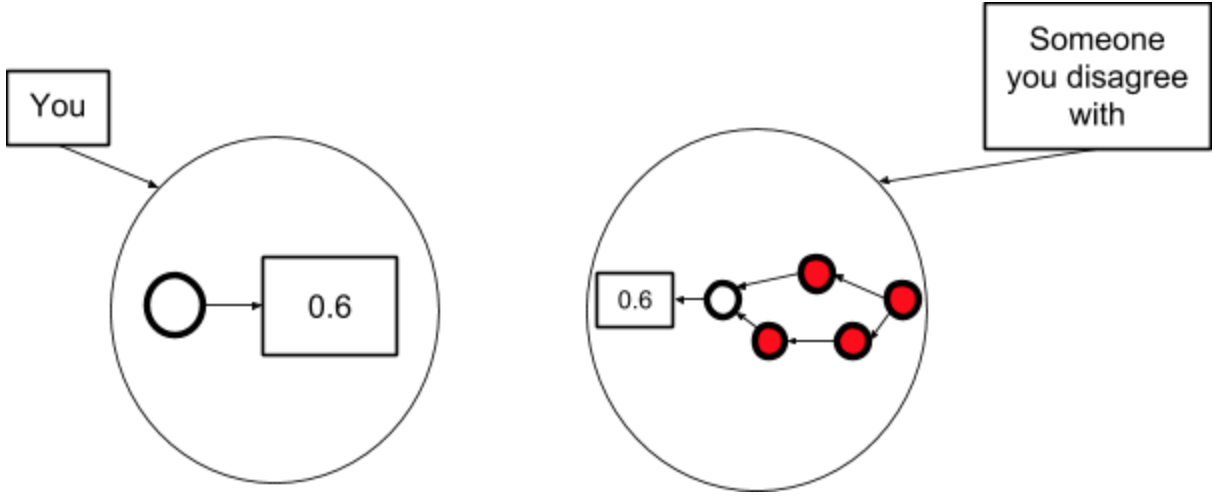
This last one is the worst, because it means you can’t notice your confusion any more. It represents “Ah, I notice that p = 0.6 is inconsistent with my model, therefore I will throw out my model.” Equivalently, “Oh, I don’t understand something, so I’ll stop trying.”
This is the first post in a series of short thoughts on epistemic rationality, integrity, and curiosity. My thanks to Jacob Lagerros and Alex Zhu for comments on drafts.
Descriptions of your experiences of successful communication about subtle intuitions (in any domain) are welcomed.
(I found your comment really clear and helpful.)
It's important to have a model of whether AlphaGo is trustworthy before you should trust it. Knowing either (a) it beat all the grandmasters or (b) its architecture and amount of compute it used, is necessary for me to take on its policies. (This is sort of the point of Inadequate Equilibria - you need to make models of the trustworthiness of experts.)
I think I'd say something like: it may become possible to download the policy network of AlphaGo - to learn what abstractions it's using and what it pays attention to. And AlphaGo may not be able to tell you what experiences it had that lead to the policy network (it's hard to communicate tens of thousands of game's worth of experience). Yet you should probably just replace your Go models with the policy network of AlphaGo if you're offered the choice.
A thing I'm currently confused on regarding this, is how much one is able to further update after downloading the policy network of such an expert. How much evidence should persuade you to change your mind, as opposed to you expecting that info to be built into the policy network you have?
You're right, it does seem that a bunch of important stuff is in the subtle, hard-to-make-explicit reasoning that we do. I'm confused about how to deal with this, but I'll say two things:
I'm reminded of the feeling when I'm processing a new insight, and someone asks me to explain it immediately, and I try to quickly convey this simple insight, but words I say end up making no sense (and this is not because I don't understand it). If I can't communicate something, one of the key skills here is acting on your models anyway, even if were you to explain your reasoning it might sound inconsistent or like you were making a bucket error.
And yeah, for the people who you think its really valuable to download the policy networks from, it's worth spending the time building intuitions that match theirs. I feel like I often look at a situation using my inner Hansonian-lens, and predict what he'll say (sometimes successfully), even while I can't explicitly state the principles Robin is using.
I don't think the things I've just said constitute a clear solution to the problem you raised, and I think my original post is missing some key part that you correctly pointed to.