I think the RLHF solves 80% of the problems of outer alignment, and I expect it to be part of the solution.
But :
- RLHF doesn't fully solve the difficult problems, which are beyond human supervision, i.e. the problems where even humans don't know what is the right way to do
- RLHF does not solve the problem of goodharting: For example there is the example of the hand which wriggles in front of the ball, without catching the ball and which fools the humans. (Imho I find this counter-example very weak, and I wonder how the human evaluators could miss this problem: it's very clear in the gif that the hand does not grab the ball).
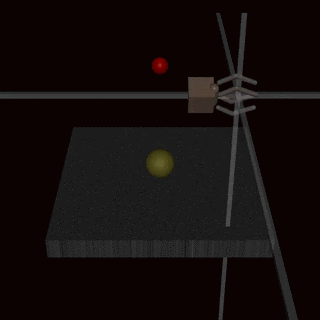
I have a presentation on RLHF tomorrow, and I can't understand why the community is so divided on this method.
RLHF is just a fancy word for reinforcement learning, leaving almost the whole process of what reward the AI actually gets undefined (in practice RLHF just means you hire some mechanical turkers and have them think for like a few seconds about the task the AI is doing).
When people 10 years ago started discussing the outer alignment problem (though with slightly different names), reinforcement learning was the classical example that was used to demonstrate why the outer alignment problem is a problem in the first place.
I don't see how RLHF could be framed as some kind of advance on the problem of outer alignment. It's basically just saying "actually, outer alignment won't really be a problem", since I don't see any principled distinction between RLHF and other standard reinforcement-learning setups.
Do you have a link for that please?