I often encounter some confusion about whether the fact that synapses in the brain typically fire at frequencies of 1-100 Hz while the clock frequency of a state-of-the-art GPU is on the order of 1 GHz means that AIs think "many orders of magnitude faster" than humans. In this short post, I'll argue that this way of thinking about "cognitive speed" is quite misleading.
The clock speed of a GPU is indeed meaningful: there is a clock inside the GPU that provides some signal that's periodic at a frequency of ~ 1 GHz. However, the corresponding period of ~ 1 nanosecond does not correspond to the timescale of any useful computations done by the GPU. For instance; in the A100 any read/write access into the L1 cache happens every ~ 30 clock cycles and this number goes up to 200-350 clock cycles for the L2 cache. The result of these latencies adding up along with other sources of delay such as kernel setup overhead etc. means that there is a latency of around ~ 4.5 microseconds for an A100 operating at the boosted clock speed of 1.41 GHz to be able to perform any matrix multiplication at all:
The timescale for a single matrix multiplication gets longer if we also demand that the matrix multiplication achieves something close to the peak FLOP/s performance reported in the GPU datasheet. In the plot above, it can be seen that a matrix multiplication achieving good hardware utilization can't take shorter than ~ 100 microseconds or so.
On top of this, state-of-the-art machine learning models today consist of chaining many matrix multiplications and nonlinearities in a row. For example, a typical language model could have on the order of ~ 100 layers with each layer containing at least 2 serial matrix multiplications for the feedforward layers[1]. If these were the only places where a forward pass incurred time delays, we would obtain the result that a sequential forward pass cannot occur faster than (100 microseconds/matmul) * (200 matmuls) = 20 ms or so. At this speed, we could generate 50 sequential tokens per second, which is not too far from human reading speed. This is why you haven't seen LLMs being serviced at per token latencies that are much faster than this.
We can, of course, process many requests at once in these 20 milliseconds: the bound is not that we can generate only 50 tokens per second, but that we can generate only 50 sequential tokens per second, meaning that the generation of each token needs to know what all the previously generated tokens were. It's much easier to handle requests in parallel, but that has little to do with the "clock speed" of GPUs and much more to do with their FLOP/s capacity.
The human brain is estimated to do the computational equivalent of around 1e15 FLOP/s. This performance is on par with NVIDIA's latest machine learning GPU (the H100) and the brain achieves this performance using only 20 W of power compared to the 700 W that's drawn by an H100. In addition, each forward pass of a state-of-the-art language model today likely takes somewhere between 1e11 and 1e12 FLOP, so the computational capacity of the brain alone is sufficient to run inference on these models at speeds of 1k to 10k tokens per second. There's, in short, no meaningful sense in which machine learning models today think faster than humans do, though they are certainly much more effective at parallel tasks because we can run them on clusters of multiple GPUs.
In general, I think it's more sensible for discussion of cognitive capabilities to focus on throughput metrics such as training compute (units of FLOP) and inference compute (units of FLOP/token or FLOP/s). If all the AIs in the world are doing orders of magnitude more arithmetic operations per second than all the humans in the world (8e9 people * 1e15 FLOP/s/person = 8e24 FLOP/s is a big number!) we have a good case for saying that the cognition of AIs has become faster than that of humans in some important sense. However, just comparing the clock speed of a GPU to the synapse firing frequency in the human brain and concluding that AIs think faster than humans is a sloppy argument that neglects how training or inference of ML models on GPUs actually works right now.
While attention and feedforward layers are sequential in the vanilla Transformer architecture, they can in fact be parallelized by adding the outputs of both to the residual stream instead of doing the operations sequentially. This optimization lowers the number of serial operations needed for a forward or backward pass by around a factor of 2 and I assume it's being used in this context. ↩︎
Yes if you use 1 die with 1 clock domain, they would. Modern chips don't.
So actually you would make something like 10,000 dies, the MAC units spread between them. Clusters of dies are calculating a single layer of the network. There are many optical interconnects between the modules, using silicon photonics, like this: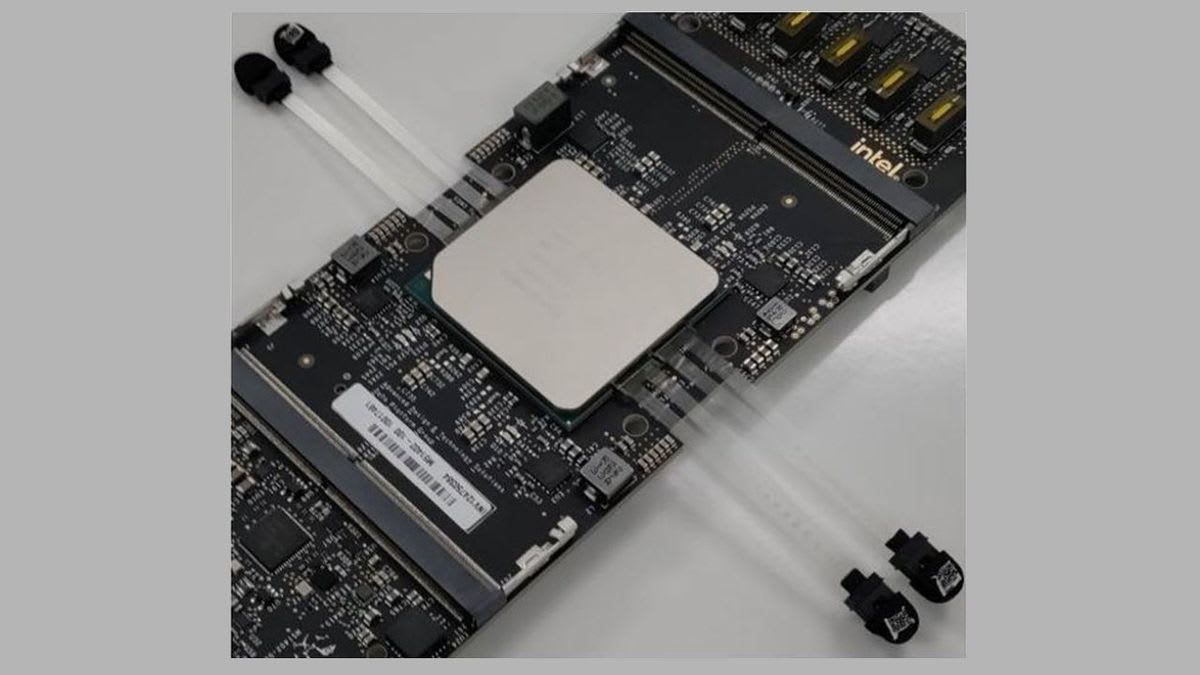
I was focused on the actual individual synapse part of it. The key thing to realize that the MAC is the slowest step, multiplications are complicated and take a lot of silicon, and I am implicitly assuming all other steps are cheap and fast. Note that it's also a pipelined system with enough hardware there aren't any stalls, the moment a MAC is done it's processing the next input on the next clock, and so on. It's what hardware engineers would do if they had an unlimited budget for silicon.
No no no. Each layer runs 1 million times faster. If a human brain needs 10 seconds to think of something, that's enough time for the AI equivalent to compute the activations of 10 million layers, or many forward passes over the information, reflection on the updated memory buffer from the prior steps, and so on.
Or the easier way to view it is : say a human responds to an event in 300 ms.
Optic nerve is 13 m/s. Average length 45 mm. 3.46 milliseconds to reach the visual cortex.
Then for a signal to reach a limb, 100 milliseconds. So we have 187 milliseconds for neural network layers.
Assume the synapses run at 1 khz total, so that means in theory there can be 187 layers of neural network between the visual cortex and the output nerves going to alimb.
So to run this 1 million times faster, we use hollow core optical fibers, and we need to travel 1 meter total of cabling. That will take 3.35 nanoseconds, which is 89 million times faster.
Ok, can we evaluate a 187 layer neural network 1 million times faster than 187 milliseconds?
Well if we take 1 clock for the MACs, we compute the activations with 9 clocks of delay including clock domain syncs, you need 10 ghz for 1 million fold speedup.
But oh wait, we have better wiring. So actually we have 299 milliseconds of budget for 187 neural network layers.
This means we only need 6.25 ghz. Or we may be able to do a layer in less than 10 clocks.
There is nothing speculative or novel here about the overall idea of using ASICs to accelerate compute tasks. The reason it won't be done this way is because you can extract a lot more economic value for your (silicon + power consumption) with slower systems that share hardware.
I mean right now there's no value at all in doing this, the AI model is just going to go off the rails and be deeply wrong within a few minutes at current speeds, or get stuck in a loop it doesn't know it's in because of short context. Faster serial speed isn't helpful.
In the future, you have to realize that even if the brain of some robotic machine can respond 1 million times faster, physics do not allow the hardware to move that fast, or the cameras to even register a new frame by accumulating photons that fast. It's not a useful speed. It also applies similarly to R&D tasks - at much lower speedups the task will be limited by the physical world.