Artificial general intelligence is often assumed to improve exponentially through recursive self-improvement, resulting in a technological singularity. There are hidden assumptions in this model which should be made explicit so that their probability can be assessed.
Let us assume that:
- The Landauer limit holds, meaning that:
- Reversible computations are impractical
- Minimum switching energy is of order J per operation
- Thus, energy cost at of order 1 EUR per FLOPs (details)
- General intelligence scales sublinear with compute:
- Making a machine calculate the same result in half the time costs more than twice the energy:
- Parallelization is never perfect (Amdahl's law)
- Increasing frequency results in a quadratic power increase ()
- Similarly, cloning entire agents does not speed up most tasks linearly with the number of agents ("You can't produce a baby in one month by getting nine women pregnant.")
- Improving algorithms will have a limit at some point
- Making a machine calculate the same result in half the time costs more than twice the energy:
My prior on (1) is 90% and on (2) about 80%.
Taken together, training ever larger models may become prohibitively expensive (or financially unattractive) for marginal gains. As an example, take an AGI with an intelligence level of 200 points, consuming 1 kW of power. Increasing its intelligence by a few points may come at 10x the power requirement. Mock visualization:
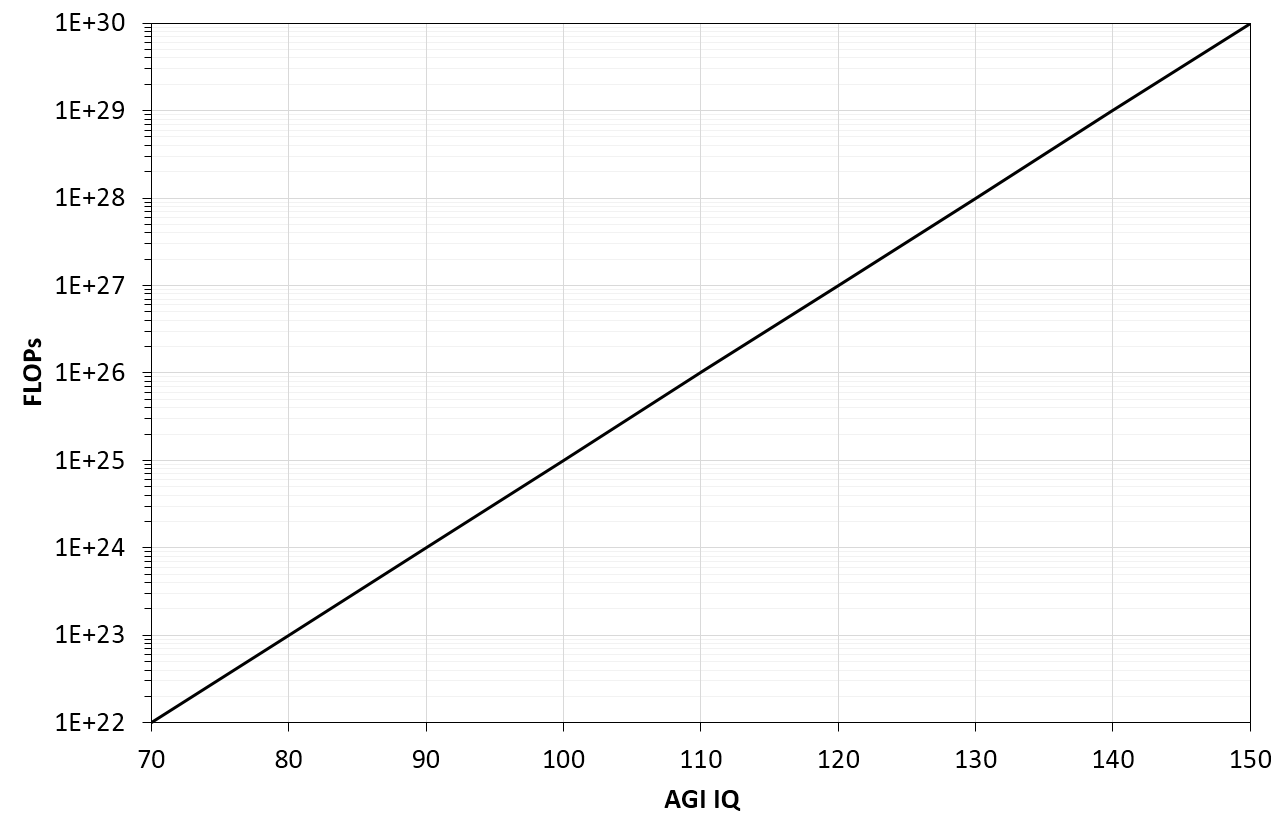
If these assumptions hold, then the exponential increase in capabilities would likely break down before a singularity is reached.
How can we know that "it is possible to train a 200 IQ equivalent intelligence for at most 3 MW-hr"?