This post relies on an understanding of two concepts: The Orthogonality thesis and the sharp left turn. If you already know what they are, skip to the main text.
The orthogonality thesis states that an agent can have any combination of intelligence and goals. It is one of the core assumptions of alignment research.
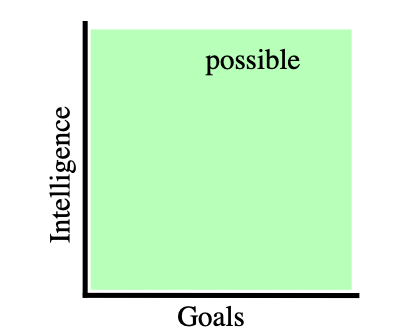
The sharp left turn is a hypothesized event, where the capabilities of an AI suddenly generalize to new domains without its alignment capabilities generalizing. This process is sometimes described as “hitting the core of intelligence” and is considered to be the crucial point of alignment by some, as AIs after a sharp left turn might be more capable than humans. So we have to have AI alignment figured out before an AI takes the sharp left turn.
Main text
While I do think the orthogonality thesis is mostly correct, I have a small caveat:
For an AI to maximize/steer towards x, x must be either part of its sensory input or its world model.
Imagine a really simple “AI”: a thermostat that keeps the temperature. If you take a naive view of the orthogonality thesis, you would have to believe that there is a system as intelligent as a thermostat, that maximizes paperclips. I do not think there is such a system, because it would have no idea what a paperclip is. It doesn't even have a model of the outside world, it can only directly act on its sensory input.
Even for systems that have a world model, they can only maximize things that are represented in their world model. If that world model only includes objects in a room, but the AI does not have the concept of what a person is, and what intentions are, it can optimize towards “put all the red squares in one line” but it can not optimize towards “do what the person intends”.
So a more realistic view of the orthogonality thesis is the following:
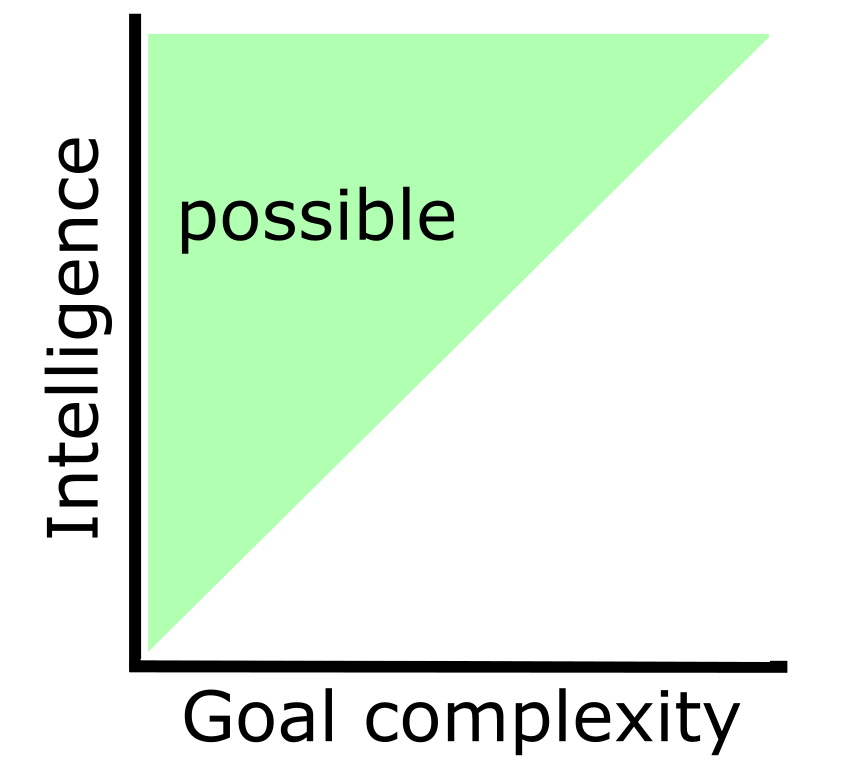
In this plot, I have lumped intelligence and world model complexity together. Usually, these concepts go together, because the more intelligent an AI is, the more complex its world model gets. If we found a way to make an AI have an arbitrarily complex world model while still being arbitrarily dumb, this graph would no longer hold true.
Now, this is where the sharp left turn comes into play. Assuming that there is a sharp left turn, there is some maximum intelligence that a system can have, before “hitting the core of intelligence” and suddenly becoming stronger than humans.
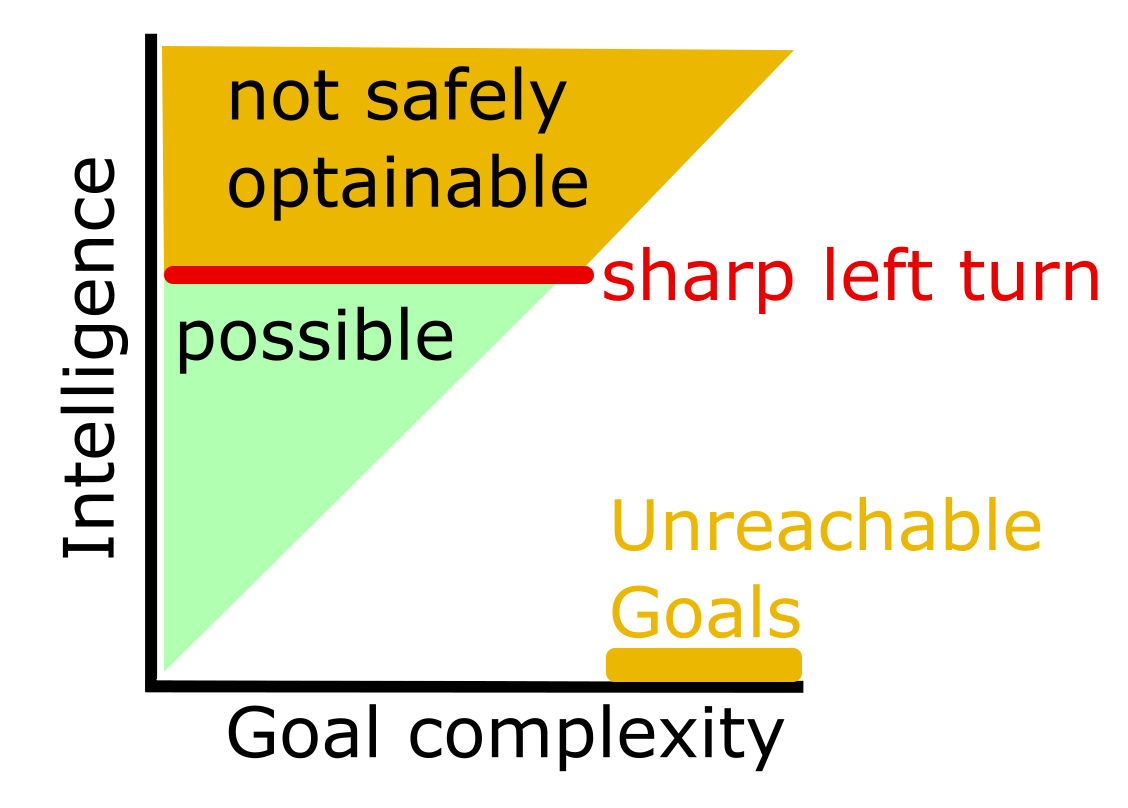
The important question is, whether a system hits this core of intelligence just by having a more and more sophisticated world model. If this is the case, then there is some maximum amount of world model complexity that a system can have before taking the sharp left turn. And that would mean in turn, that there is a cap on the goals that we can give an AI in practice.
There might be Goals that are so complex, that any AI intelligent enough to understand them, would have hit the sharp left turn.
There are follow up questions, that I have no answers to, and would be grateful for insightful comments:
- Is it true that a more and more sophisticated world model is enough to “hit the core of intelligence”?
- Are human values in the reachable or the unreachable goals?
- Is corrigibility in the reachable or the unreachable goals?
The sharp left turn argument boils down to some handwavey analogy that evolution didn't optimize humans to optimize for IGF - which is actually wrong, as it clearly did, combined with another handwavey argument that capabilities will fall into a natural generalization attractor, but there is no such attractor for alignment. That second component of the argument is also incorrect, because there is a natural known attractor for alignment - empowerment. The more plausible attractor argument is that selfish-empowerment is a stronger attractor than altruistic-empowerment.
Also more generally capabilities generalize through the world model, but any good utility function will also be defined through the world model, and thus can also benefit from its generalization.
Not quite - if I was saying that I would have. Instead I'd say that as the world model improves through training and improves its internal compression/grokking of the data, you can then also leverage this improved generalization to improve your utility function (which needs to reference concepts in the world model). You sort of have to do these updates anyway to not suffer from "ontologi... (read more)