Blake Richards is a neuroscientist / AI researcher with appointments at McGill & MiLA. Much of his recent work has involved making connections between machine learning algorithms and the operating principles of the cortex and hippocampus, including theorizing about how the neocortex might accomplish something functionally similar to backprop. (Backprop itself is not biologically plausible.) I have read lots of his papers; they're always very interesting!
Anyway, Blake recently gave a nice interview on Michaël Trazzi’s podcast “The Inside View”. Episode and transcript at: https://theinsideview.ai/blake.
Blake said a lot of things in the interview that I wholeheartedly agree with; for example, I would echo pretty much everything Blake said about scaling. But Blake & I strongly part ways on the subject of (what I call) artificial general intelligence (AGI). (Blake doesn’t like the term “AGI”, more on which below.) So that’s what I’ll talk about in this post.
1. Two mental images for thinking about an AI. Or: My lips say “human-level”, but my heart says “a fancier version of GPT or AlphaFold”
I’ll jump right into a key issue that I think is lurking below the surface. Here are two mental images for thinking about an AI system (I’ll be returning to this table throughout the post)
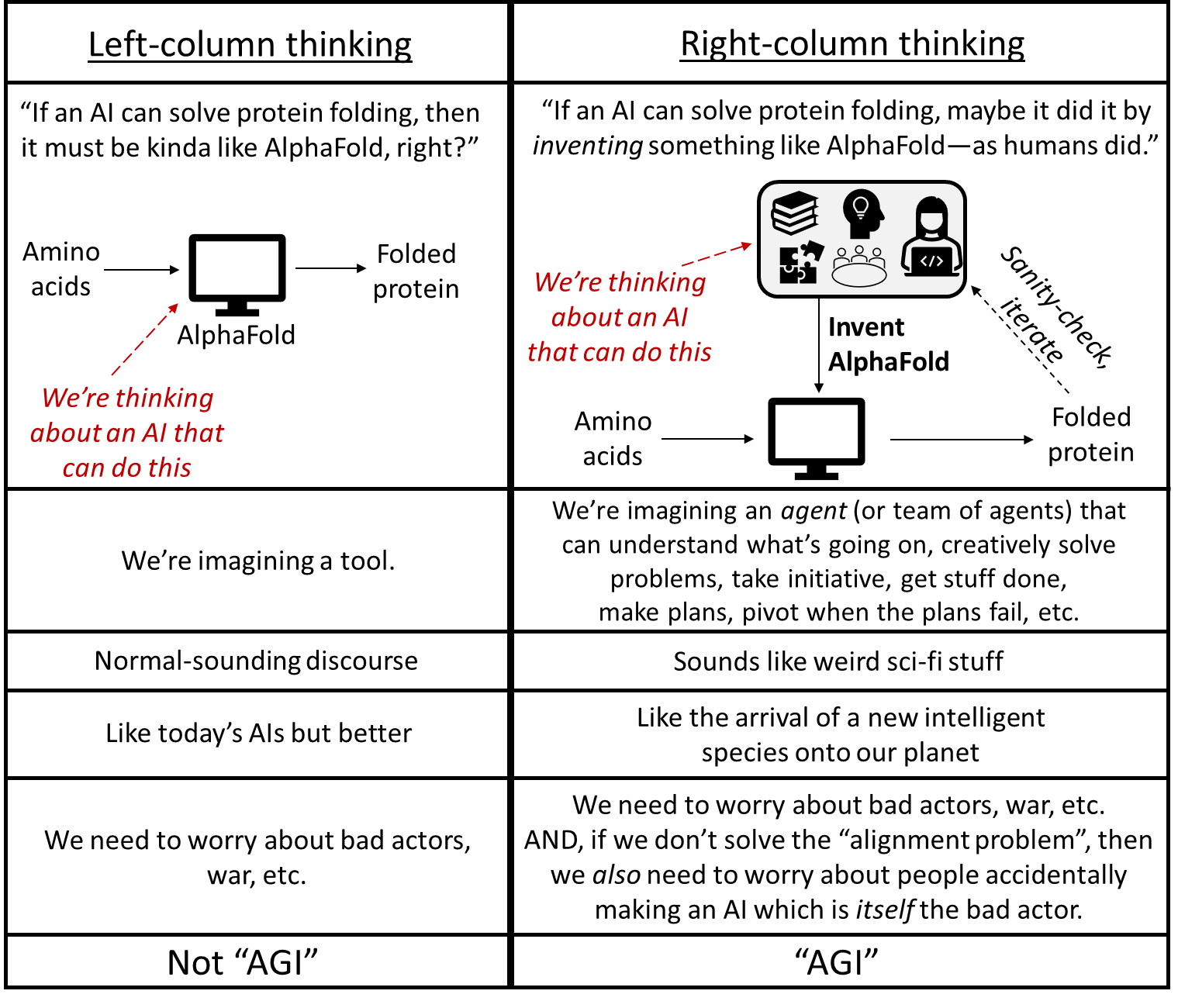
Throughout the interview, even while Blake was saying things like “as general as a human”, I got a strong feeling that what he was imagining was the thing in the left column. But if it was actually as general as a human, it would look like the thing in the right column. For example, if an AI is actually as general as a (very competent) human, and you ask it to fold proteins, and it doesn’t know how, then it downloads AlphaFold. Or if AlphaFold doesn’t exist, it starts reading papers and textbooks, and goes about inventing AlphaFold!
(In Section 3 below, I’ll go over an example of one of the many things Blake said, in the interview, that gave me the strong impression that he was engaging in left-column thinking. If I’m wrong, then so much the better—we can just skip to the next section!)
Anyway, left-column thinking is extremely natural, because that’s what ML looks like today. But we know that it’s technically possible to make AIs that require right-column thinking—Blake and I are in full agreement that anything the human brain can do, an AI can do too.
A further question is, even if the right-column AIs are technically possible, will humans actually create them? It seems to me that we should have quite high credence on “Yes”. After all, how do you prevent anyone, anywhere on earth, from solving a scientific problem and implementing the corresponding algorithm, forever and ever?? Remember when the USA tried to ban people from talking about a certain algorithm? It was not a permanent solution, to say the least! And of course, Blake himself is one of the many people devoting their professional lives to knocking down the technical barriers that prevent us from building right-column AIs.
Thus, it seems to me that we will, sooner or later, have right-column AIs. And those future AIs are the thing that I want to talk about in this post.
2. We need a term for the right-column thing, and “Artificial General Intelligence” (AGI) seems about as good as any.
That brings us to the term "AGI" for talking about the thing in the right column of the table above. Blake (like Yann LeCun) does not like the term “AGI”, but I’m going to use it anyway in this post, and here I’ll explain why.
The thing I feel strongest about is that we need some term—any term—for the thing in the right column. Because it’s a thing we need to talk about. I don’t feel very strongly that this term has to be “AGI” in particular. But something.
Consider: If all organisms have a “niche”, then the “human niche” includes living on every continent, walking on the moon, inventing computers and nuclear weapons, and unraveling the secrets of the universe. What happens when we make AI systems that can occupy that same niche? Think about AI systems that can do literally every aspect of my job. Think about AI systems which, when motivated to invent a better solar cell, are able to think to themselves, “Y'know, I'd be able to proceed much faster if I had more compute for my semiconductor simulations. Can I rent some? Oh, I need money. What if I steal it?” What happens if and when we bring these systems into the world?
This is an important conversation, and in order to have that conversation, a prerequisite is to have a jargon term with which we can refer to right-column AI systems. And the most common such term is “Artificial General Intelligence” (“AGI”).
I happen to think that “AGI” is a perfectly fine term to refer to this thing, as long as it's understood that:
- The G is “general as in not specific” (“in general, Boston has nice weather”).
- The G is NOT “general as in universal” (“I have a general proof of the math theorem”).
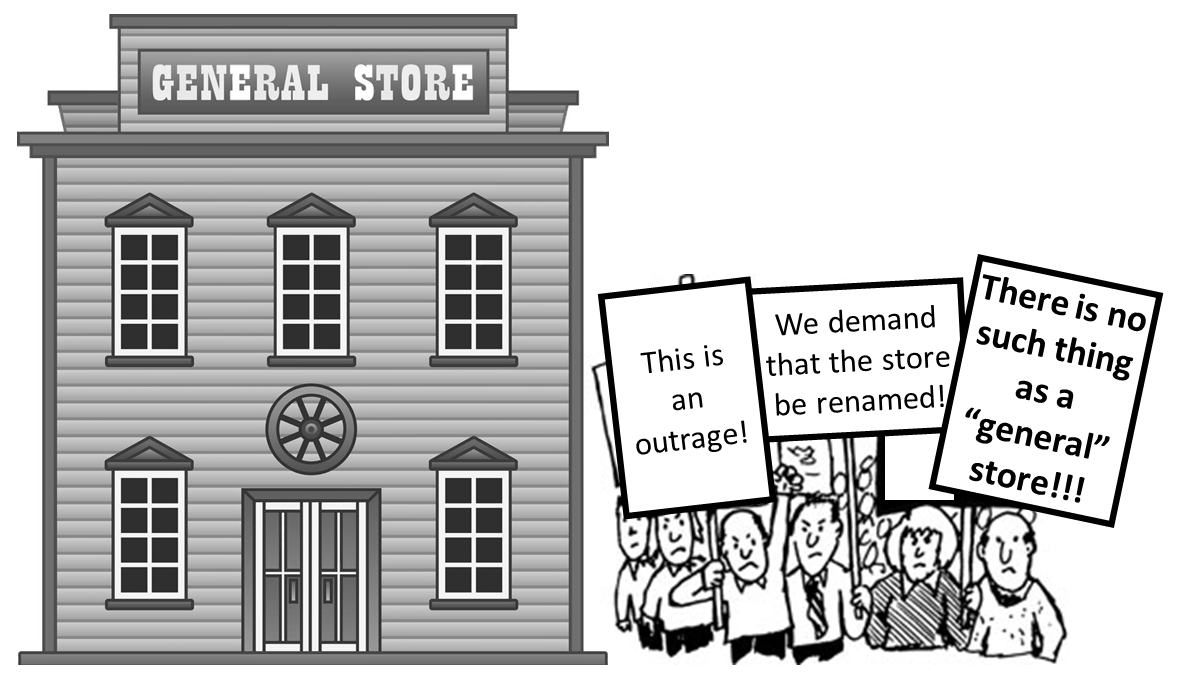
Other people don’t like the term “AGI”, and talk about the same concept using a different term. It seems to me that none of the options is particularly great:
- I believe Yann LeCun likes the term “human-level machine intelligence”. But then that creates a different set of confusions, which require a different set of clarifications, like “…but it could think 1000× faster than a human”, and “…but it may lack a human-level sense of smell”.
- Eliezer Yudkowsky uses “AGI”, but suggests that we treat “general intelligence” as an abbreviation for “Significantly More Generally Applicable Intelligence”. (If only “SMGAI” were more catchy!)
- “Transformative AI” is somewhat related, but defined via how strongly the AI impacts the world, not whether the AI is left-column or right-column.
- “High-Level Machine Intelligence” seems OK, I guess, but in practice seems a bit obscure; when I say that term, nobody knows what I'm talking about, whereas I find most people understand the term “AGI” to mean approximately what I intend it to mean.
For the purpose of this post, following common usage, I’ll use “AGI” to talk about that thing in the right column above. If that bothers you, you can use a browser extension to replace “AGI” with the term of your choice. ¯\_(ツ)_/¯
Incidentally, I don’t claim that there’s a sharp line between the “AIs” in the left column, and the “AGIs” in the right column. But there are systems that are definitely in the right column, and for reasons described above, I expect that someday we will build them.
3. Blake’s “specialization” argument against AGI
Here’s an excerpt from Blake’s argument on the podcast:
We know from the no free lunch theorem that you cannot have a learning algorithm that outperforms all other learning algorithms across all tasks. It’s just an impossibility. So necessarily, any learning algorithm is going to have certain things that it’s good at and certain things that it’s bad at. Or alternatively, if it’s truly a Jack of all trades, it’s going to be just mediocre at everything. Right? So with that reality in place, you can say concretely that if you take AGI to mean literally good at anything, it’s just an impossibility, it cannot exist. And that’s been mathematically proven.
Now, all that being said, the proof for the no free lunch theorem, refers to all possible tasks. And that’s a very different thing from the set of tasks that we might actually care about. Right?
Because the set of all possible tasks will include some really bizarre stuff that we certainly don’t need our AI systems to do. And in that case, we can ask, “Well, might there be a system that is good at all the sorts of tasks that we might want it to do?” Here, we don’t have a mathematical proof, but again, I suspect Yann’s intuition is similar to mine, which is that you could have systems that are good at a remarkably wide range of things, but it’s not going to cover everything you could possibly hope to do with AI or want to do with AI.
At some point, you’re going to have to decide where your system is actually going to place its bets as it were. And that can be as general as say a human being. So we could, of course, obviously humans are a proof of concept that way. We know that an intelligence with a level of generality equivalent to humans is possible and maybe it’s even possible to have an intelligence that is even more general than humans to some extent. I wouldn’t discount it as a possibility, but I don’t think you’re ever going to have something that can truly do anything you want, whether it be protein folding, predictions, managing traffic, manufacturing new materials, and also having a conversation with you about your [grandma’s] latest visit that can’t be… There is going to be no system that does all of that for you.
3.1 “Something that can truly do anything you want” is less crazy than it initially sounds, under right-column thinking
Again, here’s Blake in the interview:
…I don’t think you’re ever going to have something that can truly do anything you want, whether it be protein folding, predictions, managing traffic, manufacturing new materials, and also having a conversation with you about your [grandma’s] latest visit…
Now let’s try this modification, where we switch from left-column thinking to right-column thinking:
…I don’t think you’re ever going to have something that can truly do anything you want, whether it be building a system for protein folding, building a system for predictions, building a system for managing traffic, building a system for manufacturing new materials, and also building a system for having a conversation with you about your [grandma’s] latest visit…
Now it’s not so obvious that such an AI can’t exist, right?
For example, let’s take young adult John von Neumann, give him complete access to the ML and biology and chemistry and physics literature, and a compute cluster, and 30 years to work on the problem. Could he build a state-of-the-art system that takes amino acids and calculates folded proteins? I dunno! But maybe! It hardly seems an outlandish thing to expect.
OK, next, let’s take the same young adult John von Neumann, give him complete access to the ML and robotics and transportation engineering literature, and a compute cluster, and a robotics lab, and money to hire technicians, and 30 years to work on the problem. Could he build a state-of-the-art robot traffic guard? Again, I dunno! But maybe!
Now take the same young adult John von Neumann, give him complete access to the chemistry and chemical engineering and finance and management literature, along with a big pot of money to buy land and hire people, and 30 years to work on the problem. Could he build a state-of-the-art factory for manufacturing new materials? Maybe!
You get the idea.
Anyway, I grant that expertise in the downstream tasks here (folding proteins, directing traffic, producing chemicals) would not seem to have any overlap. But expertise in the upstream tasks (figuring out what kind of system would solve these tasks, and then building it) seem like they have quite a bit in common. For example, just go ask any successful startup founder to list everything they’ve had to figure out how to do.
And whatever is happening in John von Neumann’s brain that enables him to figure out how to do all those different things, we can make an AI that can run all the same calculations, and thus figure out all those same things.
3.2 Cranking up our example beyond John von Neumann
As impressed as we should be by John von Neumann’s ability to figure things out, this is not by any means the limit of what we should expect from future AIs. Here are four examples of how an AI can do better:
First, cloning. Future AGIs will be able to instantly clone themselves. So if our John von Neumann AGI has its hands full doing chemical simulations, it can spin off a whole team of copies, and one can start hiring employees, while another starts picking a plot of land, and so on. See The Duplicator: Instant Cloning Would Make the World Economy Explode.
Second, teams more generally. Maybe you think it’s eternally beyond John von Neumann’s capability to figure out some particular thing—let’s say, how to write good advertising copy. It’s just not what his brain is good at.
If that’s what you think, then you’re slipping back into left-column thinking!
In fact, if our John-von-Neumann-like AGI needs good advertising copy, and can’t figure out how to create it, then he could go ahead and train up a David-Oglivy-like AGI and ask it for help!
Actually, Blake brought this up in his interview:
To some extent you could argue that our society at large is like that, right? … Some people who are specialized at art, some people who are specialized at science and we call upon those people differently for different tasks. And then the result is that the society as a whole is good at all those things, to some extent. So in principle, that’s obviously a potential strategy. I think an interesting question that way is basically, “To what extent you would actually call that an AGI?”
My answer to the last question is: “Yes I would absolutely 100% call that an AGI”.
I think this is another instance of left-column-thinking versus right-column-thinking. In my (right-column) book, an AI that can call upon a wide variety of tools and resources (including other AIs), as the situation demands—and which can also go find and build more tools and resources (and AIs) when its existing ones are insufficient—is exactly the prototypical thing that we should be thinking of when we hear the word “AGI”!
So consider the entire human R&D enterprise—every human, at every university, every private-sector R&D lab, every journal editor, etc. This enterprise is running hundreds of thousands of laboratory experiments simultaneously, it’s publishing millions of papers a year, it’s synthesizing brilliant new insights in every field all at once. A single future AGI could do all that. Seems pretty “general” to me, right?
Third, speed. I expect that sooner or later, we’ll have an AGI that can not only figure out anything that John von Neumann can figure out, but that can “think” 100× faster than he could—such that normal humans look like this video. After all, we’re working with silicon chips that are 10,000,000× faster than the brain, so we can get a 100× speedup even if we’re a whopping 100,000× less skillful at parallelizing brain algorithms than the brain itself.
Fourth, “quality”. As discussed above, I claim that there’s a core set of right-column capabilities that we can loosely describe as “understanding problems, and designing systems that can solve them, including by inventing new technology”. Just as an adult John von Neumann clone is much “better” at these core capabilities than I am, I imagine that there are future AGI algorithms that would be far “better” at these capabilities than John von Neumann (or any human) would be. Not just faster, but actually better!
For example, given our finite number of cortical neurons, there’s only so much complexity of an idea that can be held in working memory. Beyond that, pieces need to be chunked and cached and explored serially, at some cost in the ability to notice connections (a.k.a. “insight”). Humans only have so many cortical neurons, but we can (and, I presume, eventually will) make an AGI that runs neocortex-like algorithms, but with a factor-of-100 scale-up (with appropriately adjusted architecture, hyperparameters, etc.).
Practically every time we try scaling up ML algorithms, we wind up surprised and impressed by the new capabilities it unlocks. I don’t see why that wouldn’t be true for the neocortex learning algorithm too.
(And I won’t even talk about the whole universe of possible algorithms that are more drastically different than the neocortex.)
Summary: Even if you discard the more speculative fourth point, I conclude that we should absolutely expect future AI systems that any reasonable person would describe as “radically superintelligent”, and that can trounce any human in any cognitive task just as soundly as AlphaZero can trounce humans in Go.
4. AGI accidents and the alignment problem
Here’s Blake again on the podcast:
I think if anything, what I fear far more than AI doing its own bad thing is human beings using AI for bad purposes and also potentially AI systems that reflect other bad things about human society back to us and potentially amplify them. But ultimately, I don’t fear so much the AI becoming a source of evil itself as it were. I think it will instead always reflect our own evils to us.
First I need to address the suggestion that there’s an either/or competition between worrying about out-of-control-AGI accidents versus worrying about AI misuse and related issues. Then I’ll talk about the former on its own terms. I won’t dive into the latter in this post, because it seems that Blake and I are already in agreement on that topic: we both think that there are real and serious problems related to AI misuse, weaponization, surveillance, exacerbation of inequality, and so on.
4.1 The out-of-control-AGI-accidents problem can be a real problem, and the AI misuse problem can also simultaneously be a real problem. It’s not either/or!
In addition to the quote above, here’s Blake on Twitter:
I find it kinda funny to worry about the threat of super intelligent AI in the future when we face the much more immediate and real threat of dumb AI with super power over people's lives.
And in replies:
…There is a fourth possibility, which motivated my tweet:
The probability of super intelligence this century is sufficiently low, and the probability of irresponsible use of dumb AI is sufficiently high, that the latter is much more worrying than the former.
I think half of the appeal of hand-wringing over super intelligent AI is precisely that it allows one (particularly tech ppl) to avoid asking hard questions about the here-and-now.
Now consider the following:
- Suppose I give a lecture and say: “Why worry about the risks of future sea level rise? People are dying of heart disease right now!!!” I think everyone would look at me like I was nuts.
- Suppose I give a lecture and say: “Why worry about the risks of future superintelligent AI? We have narrow AI poisoning the political discourse right now!!!” I think the audience would nod solemnly, and invite me to write a New York Times op-ed.
But what the heck?? It’s the same argument! (For more on this, see my later post: “X distracts from Y” as a thinly-disguised fight over group status / politics)
More explicitly: a priori, the various problems arising from dumb AI might or might not be real problems—we need to figure it out. Likewise, a priori, the problem of future hyper-competent misaligned AGIs getting out of control and wiping out humanity might or might not be a real problem—we need to figure it out. But these are two different topics of investigation. There’s no reason to view them as zero-sum.
If anything, we should expect a positive correlation between the seriousness of these two problems, because they do in fact have a bit of structural overlap. As one of many examples, both social media recommendation algorithm issues and out-of-control-AGI issues are exacerbated by the fact that huge trained ML models are very difficult to interpret and inspect. See The Alignment Problem by Brian Christian for much more on the overlap between near-term and long-term AI issues.
Anyway, there are many a priori plausible arguments that we shouldn’t worry about superintelligent AGI. I’m just claiming that the existence of AI misuse is not one of those arguments, any more than the existence of heart disease is.[1] Instead, we need to just talk directly about superintelligent AGI, and address that topic on its merits. That brings us to the next section:
4.2 The AGI alignment problem: not only a real problem, but a problem that Blake himself is unusually well suited to work on!
So, how about superintelligent AGI? When might it arrive (if ever)? What consequences should we expect? Isn’t it premature to be thinking about that right now?
For the long answer to these questions, I will shamelessly plug my blog-post series Intro to Brain-Like AGI Safety! Here’s the summary:
- We know enough neuroscience to say concrete things about what “brain-like AGI” would look like (Posts #1–#9);
- In particular, while “brain-like AGI” would be different from any known algorithm, its safety-relevant aspects would have much in common with actor-critic model-based reinforcement learning with a multi-dimensional value function (Posts #6, #8, #9);
- “Understanding the brain well enough to make brain-like AGI” is a dramatically easier task than “understanding the brain” full stop—if the former is loosely analogous to knowing how to train a ConvNet, then the latter would be loosely analogous to knowing how to train a ConvNet, and achieving full mechanistic interpretability of the resulting trained model, and understanding every aspect of integrated circuit physics and engineering, etc. Indeed, making brain-like AGI should not be thought of as a far-off sci-fi hypothetical, but rather as an ongoing project which may well reach completion within the next decade or two (Posts #2–#3);
- In the absence of a good technical plan for avoiding accidents, researchers experimenting with brain-like AGI algorithms will probably accidentally create out-of-control AGIs, with catastrophic consequences up to and including human extinction (Posts #1, #3, #10, #11);
- Right now, we don’t have any good technical plan for avoiding out-of-control AGI accidents (Posts #10–#14);
- Creating such a plan seems neither to be straightforward, nor to be a necessary step on the path to creating powerful brain-like AGIs—and therefore we shouldn’t assume that such a plan will be created in the future “by default” (Post #3);
- There’s a lot of work that we can do right now to help make progress towards such a plan (Posts #12–#15);
- There is funding available to do this work (Post #15).
So check out that series for my very long answer.
For a much shorter answer, I would turn to Blake’s own research. As far as I understand, Blake holds the firm belief that the right starting point for thinking about the cortex is that the cortex runs a within-lifetime learning algorithm, adjusting parameters to minimize some loss function(s). I strongly agree, and would go further by claiming that well over 90% of human brain volume (including the whole cortex [including hippocampus], striatum, amygdala, and cerebellum) is devoted to running within-lifetime learning algorithms, whereas the other <10% (mainly brainstem & hypothalamus) are more-or-less hardcoded by the genome. (See Post #2 of my series for various details and caveats on that.)
Blake and his students and colleagues spend their days trying to understand the nuts and bolts of the brain's various within-lifetime learning algorithms.
The question I would pose is: What if they succeed? What if they and others eventually come to understand the operating principles of the brain’s within-lifetime learning algorithms (and associated neural architecture etc.)—maybe not every last detail, but enough to build ML models that aren’t missing any big critical learning or inference tricks that the brain uses. Then what?
Then I claim there would be essentially nothing stopping future researchers from building real-deal right-column human-level AGI. (Some caveats on that here and here.)
But would it be human-like AGI, with human-like inclinations to friendship, norm-following, laziness, tribal loyalty, etc.? No! Not unless those learning algorithms also had human-like loss functions![2]
Recall, a funny thing about learning algorithms is that they can be pointed at almost anything, depending on the loss function. With one reward function, AlphaZero learns to play chess; with a different reward function, AlphaZero would learn to play suicide chess—achieving the exact opposite goal with superhuman skill.
In humans, I claim that “loss functions”[2] loosely correspond to “innate drives” (details here), and are intimately related to circuitry in the hypothalamus and brainstem (i.e. the <10% of the human brain that I claim is not devoted to within-lifetime learning).
Now, when future researchers build AGIs, they will be able to put in any innate drives / loss functions that they want.
But there is no scientific theory such that you can tell me what you want an AGI to do, and then I respond by telling you what loss functions to put into your AGI, such that it will actually try to do that.
For example, I want to ask the following question:
- What loss function(s), when sent into a future AI’s brain-like configuration of neocortex / hippocampus / striatum / etc.-like learning algorithms, will result in an AGI that is trying to bring about a utopia of human flourishing, peace, equality, justice, fun, and what-have-you?
Nobody knows the answer!
…But let’s not get ahead of ourselves!! Here’s an easier question:
- What loss function(s), when sent into a future AI’s brain-like configuration of neocortex / hippocampus / striatum / etc.-like learning algorithms, will result in an AGI that is definitely not trying to literally exterminate humanity?
That’s a much lower bar! But we don’t know the answer to that one either!! (And it’s much trickier than it sounds—see this post.)
OK, here’s another question, indirectly related but probably more tractable.
- Neurotypical humans have a bunch of social instincts—friendship, envy, status drive, schadenfreude, justice, and so on. These instincts are cross-cultural universals, and therefore presumably arise from genetically-hardcoded innate drives, giving rise to corresponding loss functions. What are those loss functions? And how exactly do they give rise to the familiar downstream behaviors?
See my post Symbol Grounding & Human Social Instincts for why this is a delightfully tricky puzzle. If we solve it, we would have a recipe for loss functions that make AGIs with similar moral intuitions as humans have. Granted, I've met a human or two, and that's not the most reassuring thing. (More discussion here.) But maybe it's a starting point that we can build off of.
I find (to my chagrin) that the most algorithmically-minded, AI-adjacent neuroscientists are spending their time and talent on discovering how brain loss functions affect brain learning algorithms, while basically ignoring the question of exactly what the human brain loss functions are in the first place.[2] As it happens, Blake has not only deep knowledge of the brain’s major within-lifetime learning algorithms in the telencephalon, but also past experience studying the brainstem! So I encourage him to spend some of his considerable talents going back to his roots, by figuring out what the human brain loss functions are, how they're calculated in the hypothalamus and brainstem, and how they lead to things like friendship and envy. It's a fascinating puzzle, there's funding available, and as a cherry on top, maybe he’d be helping avert the AGI apocalypse! :)
(Thanks Adam Shimi, Justis Mills, & Alex Turner for critical comments on a draft.)
- ^
One could object that I’m being a bit glib here. Tradeoffs between cause areas do exist. If someone decides to donate 10% of their income to charity, and they spend it all on climate change, then they have nothing left for heart disease, and if they spend it all on heart disease, then they have nothing left for climate change. Likewise, if someone devotes their career to reducing the risk of nuclear war, then they can’t also devote their career to reducing the risk of catastrophic pandemics and vice-versa, and so on. So tradeoffs exist, and decisions have to be made. How? Well, for example, you could just try to make the world a better place in whatever way seems most immediately obvious and emotionally compelling to you. Lots of people do that, and I don’t fault them for it. But if you want to make the decision in a principled, other-centered way, then you need to dive into the field of Cause Prioritization, where you (for example) try to guess how many expected QALYs could be saved by various possible things you can do with your life / career / money, and pick one at or near the top of the list. Cause Prioritization involves (among other things) a horrific minefield of quantifying various awfully-hard-to-quantify things like “what’s my best-guess probability distribution for when AGI will arrive?”, or “exactly how many suffering chickens are equivalently bad to one suffering human?”, or “how do we weigh better governance in Spain against preventing malaria deaths?”. Well anyway, I’d be surprised if Blake has arrived at his take here via one of these difficult and fraught Cause-Prioritization-type analyses. And I note that there are people out there who do try to do Cause Prioritization, and AFAICT they very often wind up putting AGI Safety right near the top of their lists.
I wonder whether Blake’s intuitions point in a different direction than Cause Prioritization analyses because of scope neglect? As an example of what I’m referring to: suppose (for the sake of argument) that out-of-control AGI accidents have a 10% chance of causing 8 billion deaths in the next 20 years, whereas dumb AI has 100% chance of exacerbating income inequality and eroding democratic norms in the next 1 year. A scope-sensitive, risk-neutral Cause Prioritization analysis would suggest prioritizing the former, but the latter might feel intuitively more panic-inducing.
Then maybe Blake would respond: “No you nitwit, it’s not that I have scope-neglect, it’s that your hypothetical is completely bonkers. Out-of-control AGI accidents do not have a 10% chance of causing 8 billion deaths in the next 20 years; instead, they have a 1-in-a-gazillion chance of causing 8 billion deaths in the next 20 years.” And then I would respond: “Bingo! That’s the crux of our disagreement! That’s the thing we need to hash out—is it more like 10% or 1-in-a-gazillion?” And this question is unrelated to the topic of bad actors misusing dumb AI.
[For the record: The 10% figure was just an example. For my part, if you force me to pick a number, my best guess would be much higher than 10%.]
- ^
Everyone agrees that sensory prediction error is one of the loss functions, but I’m talking about the less obvious and more safety-relevant loss functions, i.e. the ones related to “reward”, which help determine a person’s motivations / goals / drives. (See §4.7 here for why self-supervised learning is not adequate for training appropriate actions and decisions, if that isn’t obvious.)
Who else are you thinking of when you talk about "algorithmically-minded, AI-adjacent neuroscientists"? A lot of scientific research seems done nowadays online, so I'm wondering whether I need to know the relevant twitter accounts/forums/whatever if I want to keep up with this research.
Also, I feel like people misinterpret the no-free lunch theorem. (One of) the theorems states that for any algorithms A and B, there are approximately as many prior functions on which A outperforms B as vice versa. But like, we don't care about most prior functions, we care about kolmogorov complexity relative to us. So what if an AGI algorithm fails on tonnes of priors that are alien to ours?
Yeah, good point. I think I was blindly pattern matching the consecutive mentions of "no free lunch" and "can't get much better than humanity" to mean they believe the former strongly implies the latter. Probably because I don't have a good model of his views, so I just interpert them as having whatever model fits some of his statements.
Thanks for the recommendations.