https://linktr.ee/menhguin
Hello! I’ve made 2 quick improvements, mainly with prompt and tokens.
TL;DR I changed the prompt to
prompt = '<start_of_turn>user\n "<unk>"?<end_of_turn>\n<start_of_turn>model\n "<unk>" "'
I noticed that the scales were being affected by prior words in the prompt/context itself. I tried out feature 4088 and replaced some words. For example, replacing ”word”with "concept" and "number" resulted in slightly different explanations at the higher scale. Intuitively, I suspected this was a fundamental issue with “merging” 2 residual streams. So to isolate variables, I started cutting down the prompt to just:
prompt = '<start_of_turn>user\n"X"?<end_of_turn>\n<start_of_turn>model\n "X" "'
Theoretically, this reduces confounding influence between the prompt context and the SAE feature itself.
I noticed that at higher scales, explanations trended towards characters/letters specifically, despite the much shorter prompt. This was very interesting, and I realised that the explanations were picking up on the token “X” as a letter in the alphabet. So I read up on Gemma’s tokenizer to see which tokens I could replace X with. I decided on the special token <unk>, which is used to denote text that is indecipherable (I thought it would be contextually neutral, while also being appropriate for uncertainty and questioning). By combining both into this prompt:
prompt = '<start_of_turn>user\n "<unk>"?<end_of_turn>\n<start_of_turn>model\n "<unk>" "'
I’ve found explanations to be much more consistent! For example, I got subjectively much clearer results for the mission (4088), cute (4040), collection (4079) and char (14054) features across the board (see example below). I’ll keep optimising this through methods such as better entropy handling or verbosity.
Here is one example: char (14054). I picked this because it was cited as a very hard example to interpret using the current setup. You can see that prompt context removal increases direct quotation slightly, and using <unk> outputs the exact token ~80% of the time (I'll update this example later once i figure out how to format the full table).
using prompt = '<start_of_turn>user\nWhat is the meaning of the word "X"?<end_of_turn>\n<start_of_turn>model\nThe meaning of the word "X" is "'
Scale: 21.84
1. 'a amount of something; a measure". It can be used to refer to a specific amount, a quantity, a size, or a degree.\n\nFor example, the word "char" can mean'
2. 'a quantity of something; a weight". It is used as a noun or a verb.'
3. 'a specified amount or value". It is often used to indicate a fixed price or the cost of something. For example, "The price of the car is Char$ 15,000'
Scale: 27.05
1. 'a sum, total, or amount". It can be used to refer to a specific amount, a general concept, or a total quantity.\n\n* **Specific amount**: "He has charted a'
2. 'a significant amount of something, especially something precious or of high value". It can also mean "a specific amount or instance". The word can refer to material things like gold, silver, and jewels,'
3. 'a sum, total, or amount of something". It can refer to a single quantity or to the total of a set of items.'
prompt = '<start_of_turn>user\n "X" <end_of_turn>\n<start_of_turn>model\n "X" "'
Scale: 21.84
1. 'is" a common English word that means "a sum, total, or quantity of something".'
2. 'char" or "ch" can be refer to various things depending on the context. \n\n- **A letter:** The most common meaning of "char" is a single letter of the alphabet.'
3. 'is the letter in the word "charm". It is often used to indicate a positive quality or a person\'s personality.'
Scale: 27.05
1. 'is a word used to indicate a thing or a person and a possession."'
2. ' is a common English word that means "a thing, object, or idea".'
3. 'is a type of jewellery that is worn close to the body." It is often made of pearls or other gemstones.
prompt = '<start_of_turn>user\n "<unk>"?<end_of_turn>\n<start_of_turn>model\n "<unk>" "'
Scale: 21.84
1. 'Charlie", or "char" is an informal word for a person or thing that is particularly interesting, charming, or odd.'
2. 'char" are both adjectives meaning "a quantity of something". \n\n- **Char** is often used to refer to a single item, especially a piece of metal or a piece of fruit.\n'
3. 'char" or "chart" refers to a piece of something that is used for shaping, drawing, or writing.'
Scale: 27.05
1. 'is an adjective that means something that is small, light, or thin. "\n\nIt can be used to refer to a single object, or to a thing that is small in number. For example'
2. 'char" or "char" can have different meanings depending on the context:\n\n**1. Noun:**\n\n- A sheet of felt.\n- An ornament in a watch or clock.\n-'
3. 'char" or "Charlie" is a common word for a person or thing that is small or of a small size.'
I think SelfIE works about 80% as well as it could at the moment. I’ll try some other refinements:
One approach I speculated several months ago is to try and resolve the Softmax Bottleneck - Google Docs, though I’ll still look for other more tractable solutions.
I was thinking about the practical implication of this. As others have mentioned, models in production pretty much all use the prompt "you are an AI assistant". From a model training perspective, it makes sense to build with this assumption in mind.
However, it occurs to me that I have never explicitly referred to any of my AI assistants as an AI assistant. Instead, I treat them more as an inner monologue, and I suspect many other users do this as well. If the AI makes an error, I essentially correct them the way I would correct my own inner monologue/thinking in a stream of consciousness. Here, the "second brain"/"extension of the mind" paradigm is a closer example!
Could you possibly test this approach with a different kind of system prompt that focuses on inner monologues/second brains rather than assistant assumptions? It would be interesting if you can show parity or even improvement in certain tasks this way (i speculate this would improve precision in safety refusals). Would love to collaborate on this as well!
[crossposted from EA Forum, to emphasise an important point. hope that's OK! will delete if it isn't]
How do we prevent the methodology of exclusively seeking and publishing negative information, without fact checking, from becoming an acceptable norm?
Adding on as former Nonlinear intern who was aware of a “falling out” between Alice and Nonlinear for almost a year now:
I’m not against Ben and Alice choosing to post this. I believe we should normalise people exercising their option to speak out publicly. The alternative is being silenced by massive power asymmetry.
What I am against, is the way these allegations were made, which did not prioritise verifying allegations/claims when repeatedly presented with significant, factual counter-evidence.
Why was Nonlinear not given some chance to present counterevidence? It’s clear the initial investigation took months to gather; only a few days (two days, I think) before posting were Kat and Emerson presented with this, after reaching out to Ben several times! Even granting Nonlinear a day to submit an official refutation of the top 5-10 claims for review would have made a difference.[1] And that’s before factoring in the asymmetry required to refute these allegations with evidence vs making the initial allegations.
I think the handling of this community issue was not healthy for EA/longtermism. Fewer people will read this post than the initial allegations, and Nonlinear’s reputation has definitely been harmed. At best, future whistleblowers are less likely to be believed. I don’t see this as a win for anyone.
Throughout this discussion, there was this undertone that over-weighting Alice’s claims justified the increased reputational risk to Nonlinear, because Kat and Emerson are “better-off” than Alice, so harming them is a more “acceptable” risk because Kat and Emerson will still do fine, whereas Alice is new and less established in EA.
I’d like to say that these allegations don’t just affect Emerson and Kat. It affects the many independent AI Safety researchers Nonlinear helps fund.[2] It also affects Nonlinear’s other employees. It has personally affected me. I am from Southeast Asia, where it’s much harder to find work in EA/longtermism than in EA hubs. Nonlinear was the first (and currently only) EA org I’ve interned at.
Nonlinear had formally stopped hiring interns when I applied, due to the incidents mentioned above. I contributed to the Superlinear bounty platform as a remote volunteer, without knowing it was owned by Nonlinear, or what Nonlinear was. I had spent so much time trying to contribute to EA part-time, that I wanted to make the experience easier for others.
When I was hired as an intern, I texted my friend “What’s Nonlinear? Are they … like, a big deal?”. My friend explained that having Nonlinear as a reference would help me gain admission to EA conferences, and be taken seriously for EA job applications.
Now that Nonlinear’s reputation within EA has been seriously harmed, I’ve been very concerned about how this affects my ability to contribute within EA. Should I add Nonlinear/Kat as references and risk very negative associations, or omit them and risk being overlooked in favour of other applicants who do have references from prominent EAs? It means a lot to me because, as a non-US/EU/UK citizen, I know I’m always applying at a significant disadvantage.[3] I will always have fewer opportunities than an EA born in London who goes to a prestigious UK college with an active EA chapter and many EA internship options, who doesn’t have additional Visa requirements. And if I get rejected for a role, I often don’t get to know why.
I didn’t mention this before, because I cared about whether Alice was actually abused. I had a hunch they were making false claims, but I didn’t want to invalidate victims who might be telling the truth. As of now, this seems … less likely.
These allegations do cause harm: to me, to other Nonlinear employees trying to contribute to EA and the people Nonlinear helps through our work.
In the future, please verify these more seriously. Thank you.
The first time I asked Nonlinear about the allegations, it took me maybe 5-10 minutes to figure out there were multiple misleading statements, since I was shown message logs.
In fundraising, reputation matters. Serious, public allegations of abuse means funders are (rightfully) hesitant, and less funding goes to researchers.
If you are reading this and trying to get into AI Safety/longtermism from a non EA hub, do reach out and I’ll try to reply when I can! We gotta support each other >:)
Further optimisation Update Log for 28th August:
I am working from here: Minh's Copy of Gemma SAE self-explanation - Colab (google.com)
What worked: Multi-Feature Combination and Replacing Earlier Layers
Multi-feature combination works! I managed to combine feature 7656 ("France") and feature 7154 ("capital cities") from Neuronpedia's Gemma-1-2B [1] feature directory to elicit outputs for Paris, France. I'm just taking the sum of the vectors and dividing to find average, so this should work same as before even if you have 1 feature. Weighing should be relatively simple as long as you can decide how to weigh the features.
Sometimes the feature refers to regional capitals that are not Paris, or reference towns/adjectives describing towns, but that seems fair since the original capital cities feature included regional and not just national capitals (this suggests that better combination improves accuracy of desired output, which Meta SAEs does).
What didn't work: Minor Prompt Changes
I was trying to figure out how to make sentence-long prompts work. The fact that I made the prompts shorter to increase precision was great, but we were really under-leveraging the potential of complex explanation prompts:
What really really worked: Combining max activations with explanation.
Now here's the interesting part. I was (once again) trying to get anything longer than 2 words to work (it would be very cool). Here, I took inspiration from ICL literature, as well as existing autointerp methods.
Here's what I did: I looked up the mentioned features on Neuronpedia and took their max activating examples. So let's take "compile" (11866), which was considered Very Difficult To Output, even for my method.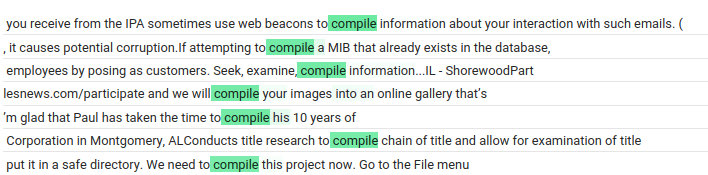
What I did was to look up the feature on Neuronpedia, replace all the activations with <unk>, and shove about 5-10 of the activating examples in the prompt.
So the prompt looks something like this[2][3].
And the model outputs are really accurate!
Example 1: "compile" (11866)
Here's "compile" (11866) - I got it to output "compile" verbatim and explain 100% of the time from scale 10-100!
Scale: 32.26
1. 'Compile" is the repeated text in the examples.\n\n"Compile" means to arrange and process the elements of a language in a systematic way, to form a code.'
2. 'Compile". In the context of these sentences, it means to compile and run a program, which generates executable code to perform a specific task.'
3. 'Compile" means to assemble or compile a piece of software or code.\n\nIn the examples given, "compile" is used to refer to the process of compiling code or software, which involves taking source'
Scale: 37.47
1. 'Compile" is used in the examples to refer to compiling an IPA, an integration process that connects and retrieves data from multiple data sources to create a unified view of a business\'s operations.'
2. 'Compile" in the context of the phrases. \n\n- "You received from the IPA sometimes use web beacons to compile information about your interaction with such emails."\n\n- "Employees by posing as customers'
3. 'Compile" is used in the examples as a synonym for "compile".\n\n"Compile" means to create a code or program from a set of instructions.\n\nIn the context of the examples, the'
Example 2: "char" (14054)
Here's "char" (14054). Repeated, and explained 100% for the time from scale 10-100!
Scale: 32.26
1. 'Char" in the examples refers to the Catholic Church. It is a reference to the charism of the charist class of religious healers, who were renowned for their fervent dedication to helping the poor and'
2. 'Char" in the context of the examples is a term used to denote a specific type of activity or person, often in the context of a person speaking prophetically or with authority.'
3. 'Char" is used in the examples to mean "charismatic", "charitable", or "charitable".'
Scale: 37.47
1. 'Char" is a synonym for "charitable". In the first example, it refers to the work of the prison ministry at Arthur Kill Correctional Facility. In the second example, it refers to the "'
2. 'Char" in the examples refers to a religious order or movement.\n\n- Her celebrity crushes are Angelina Jolie," Charismatic".\n- "Char" Renewal is a Catholic charism that promotes peace and'
3. 'Char" is used in the examples as a intensifier for adjectives like "charismatic", "charitable", "charismatic".'
For this test, I ensured that at no point is the actual output token revealed (revealing the token might be fine, I just wanted to test the limit w blind tests). What we're essentially relying on is replicating the same context as the models used in the dataset activation, and leveraging few-shot ICL to allow the models to follow instructions without losing focus on the token they're supposed to explain.
There's more tweaking to be done. But this option now allows for sentences to be used in the prompt while maintaining accuracy comparable to single-word prompts, and also makes it cross-compatible with the use of max activating examples in prior literature. I'd recommend using some combination of both anyway, since the activating examples are valuable context regardless.
I note that there's a tradeoff between specificity and open-endedness when describing particularly complex/non-obvious features. Asking specifically to describe a feature as a word would be inaccurate for a feature that isn't a word, and words are usually obvious to interpret anyway. For example, my Activating Examples method notably did not work with L12/5324 (pair of names feature), even though my "<unk>?" got the concept of describing relationships much better. It's pretty weird, I wonder why it's like that.
Remaining other stuff to try:
sometimes it's the 1-2B-IT one, honestly kinda inconsistent
yes, I know it's plaintext and not automated. I didn't have the Neuronpedia API set up and this was a quick and dirty test run.
I used {token} as a string variable so that I can easily swap out any token I want, here I'm still using <unk>