I think the RLHF solves 80% of the problems of outer alignment, and I expect it to be part of the solution.
But :
- RLHF doesn't fully solve the difficult problems, which are beyond human supervision, i.e. the problems where even humans don't know what is the right way to do
- RLHF does not solve the problem of goodharting: For example there is the example of the hand which wriggles in front of the ball, without catching the ball and which fools the humans. (Imho I find this counter-example very weak, and I wonder how the human evaluators could miss this problem: it's very clear in the gif that the hand does not grab the ball).
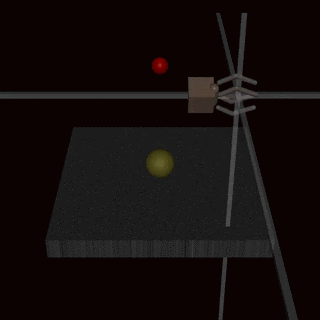
I have a presentation on RLHF tomorrow, and I can't understand why the community is so divided on this method.
Sorry, I don't understand how this is in conflict to what I am saying. Here is the relevant section from your paper:
Most mechanical turkers also have an undergraduate degree or higher, are often given long instruction manuals, and 10 minutes of thinking clearly qualifies as "thinking about a problem for a few minutes". Maybe we are having a misunderstanding around the word "problem" in that sentence, where I meant to imply that they spent a few minutes about each datapoint they provide, not like, the whole overall problem.
Scale AI used to use Mechanical Turkers (though I think they transitioned towards their own workforce, or at least filter on Mechanical Turkers additionally), and I don't think is qualitatively different in any substantial way. Upwork has higher variance, and at least in my experience doing a bunch of survey work does not perform better than Mechanical Turk (indeed my sense was that Mechanical Turk was actually better, though it's pretty hard to compare).
This is indeed exactly the training setup I was talking about, and sure, I guess you used Scale AI and Upwork instead of Mechanical Turk, but I don't think anyone would come away with a different impression if I had said "RLHF in-practice consists of hiring some random people from Upwork/Scale AI, doing some very basic filtering, giving them a 20-page instruction manual, and then having them think about a problem for a few minutes".
Oh, great! That was actually exactly what I was looking for. I had indeed missed it when looking at a bunch of RLHF papers earlier today. When I wrote my comment I was looking at the "learning from human preferences" paper, which does not say anything about rater recruitment as far as I can tell.