You can see the full voting results here: 1000+ karma voters (All voters)
The 2019 Review votes are in!
This year, 88 voters participated, evaluating 116 posts. (Of those voters, 61 had 1000+ karma, and will be weighted more highly in the moderation team's decision of what to include in the Best of 2019 Books)
The LessWrong Moderation team will be reflecting on these results and using them as a major input into "what to include in the 2019 books."
Top Results
The top 15 results from the 1000+ karma users are:
- What failure looks like by Paul Christiano
- Risks from Learned Optimization: Introduction, by evhub, Chris van Merwijk, vlad_m, Joar Skalse and Scott Garrabrant
- The Parable of Predict-O-Matic, by Abram Demski
- Book Review: The Secret Of Our Success, by Scott Alexander
- Being the (Pareto) Best in the World, by Johnswentworth
- Rule Thinkers In, Not Out, by Scott Alexander
- Book summary: Unlocking the Emotional Brain, by Kaj Sotala
- Asymmetric Justice, by Zvi Mowshowitz
- Heads I Win, Tails?—Never Heard of Her; Or, Selective Reporting and the Tragedy of the Green Rationalists, by Zack Davis
- 1960: The Year The Singularity Was Cancelled, by Scott Alexander
- Selection vs Control, by Abram Demski
- You Have About Five Words, by Raymond Arnold
- The Schelling Choice is "Rabbit", not "Stag", by Raymond Arnold
- Noticing Frame Differences, by Raymond Arnold
- "Yes Requires the Possibility of No", by Scott Garrabrant
Top Reviewers
Meanwhile, we also had a lot of great reviews. One of the most valuable things I found about the review process was that it looks at lots of great posts at once, which led me to find connections between them I had previously missed. We'll be doing a more in-depth review of the best reviews later on, but for now, I wanted to shoutout to the people who did a bunch of great review work.
The top reviewers (aggregating the total karma of their review-comments) were:
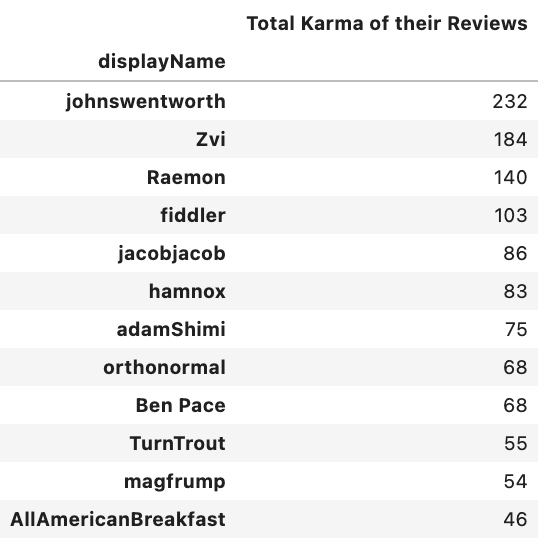
Some things I particularly appreciated were:
- johnswentworth, Zvi and others providing fairly comprehensive reviews of many different posts, taking stock of how some posts fit together.
- Jacobjacob and magfrump who stuck out in my mind for doing particularly "epistemic spot check" type reviews, which are often more effortful.
Complete Results (1000+ Karma)
You can see the full voting results here: 1000+ karma voters (All voters)
To help users see the spread of the vote data, we've included swarmplot visualizations.
- Only votes with weights between -10 and 16 are plotted. Outliers are in the image captions.
- Gridlines are spaced 2 points apart.
- Concrete illustration: The plot immediately below has 18 votes ranging in strength from -3 to 12.
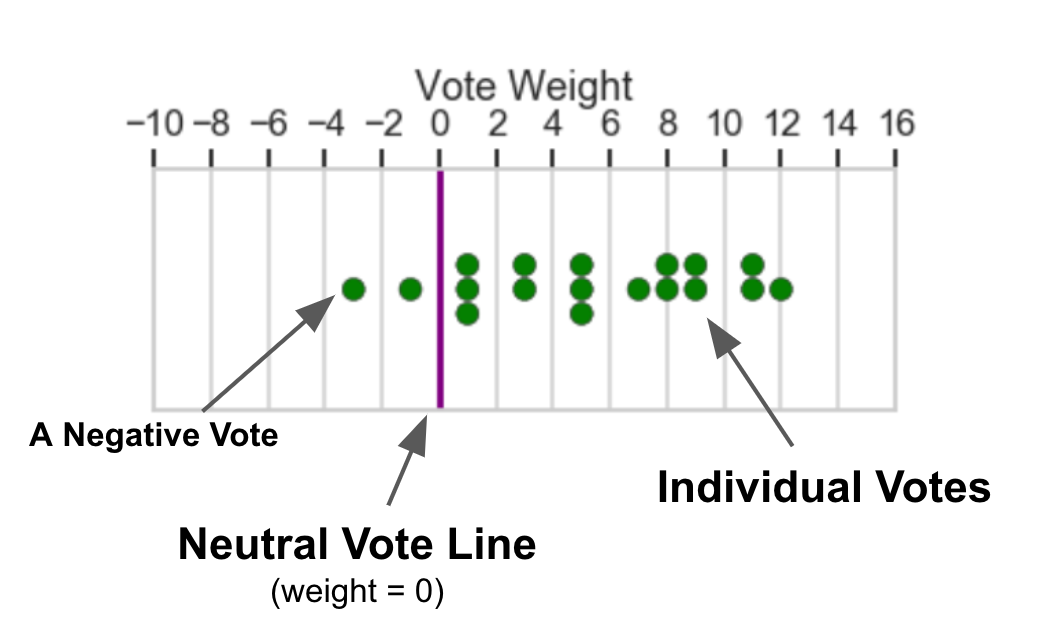
What does this mean, and what happens now?
(This section written by habryka, previous section written by Ray)
The goals of this review and vote were as follows:
- Create common knowledge about how the LessWrong community feels about various posts and the progress we've made.
- Improve our longterm incentives, feedback, and rewards for authors.
- Help create a highly curated "Best of 2019" Sequence and Book.
Over the next few months we will take the results of this vote and make it into another curated collection of essays, just as we did with last years results, which turned into the "A Map that Reflects the Territory" essay collection.
Voting, review and nomination participation was substantially greater this year than last year (something between a 30% and 80% increase, depending on which metrics you look at), which makes me hopeful about this tradition living on as a core piece of infrastructure for LessWrong. I was worried that participation would fall off after the initial excitement of last year, but I am no longer as worried about that.
Both this year and last year we have also seen little correlation with the vote results and the karma of the posts, which is an important sanity check I have for whether going through all the effort of this review is worth it. If the ranking was basically just the same as the karma scores of the post, then we wouldn't be getting much information out of the review. But as it stands, I trust the results of this review much more than I would trust someone just pressing the "sort by karma" button on the all-posts page, and I think as the site and community continues to grow, the importance of the robustness of the review will only grow.
Thank you all for participating in this year's review. I am pleased with results, and brimming with ideas for the new set of books that I am looking forward to implementing, and I think the above is already a valuable resource if someone wants to decide how to best catch up with all the great writing here on the site.
Unedited stream of thought:
Before trying to answer the question, I'm just gonna say a bunch of things that might not make sense (either because I am being unclear or being stupid).
So, I think the debate example is much more *about* manipulation, than the iterated amplification example, so I was largely replying to the class that includes IA and debate, I can imagine saying that Iterated amplification done right does not provide an incentive to manipulate the human.
I think that a process that was optimizing directly for finding a fixed point of X=AmplifyH(X) does have an incentive to manipulate the human, however this is not exactly what IA is doing, because it is only having the gradients pass through the first X in the fixed point equation, and I can imagine arguing that the incentive to manipulate comes from having the gradient pass through the second X. If you iterate enough times, I think you might effectively have some optimization juice passing through modifying the second X, but it might be much less. I am confused about how to think about optimization towards a moving target being different from optimization towards finding a fixed point.
I think that even if you only look at the effect of following the gradients coming from the effect of changing the first X, you are at least providing an incentive to predict the human on a wide range of inputs. In some cases, your range of inputs might be such there isn't actually information about the human in the answers, which I think is where you are trying to get with the automated decomposition strategies. If humans have some innate ability to imitate some non-human process, and use that ability to answer the questions, and thinking about humans does not aid in thinking about that non-human process, I agree that you are not providing any incentive to think about the humans. However, it feels like a lot has to go right for that to work.
On the other hand, maybe we just think it is okay to predict, but not manipulate, the humans, while they are answering questions with a lot of common information about humans' work, which is what I think IA is supposed to be doing. In this case, even if I were to say that there is no incentive to "manipulate the human", I still argue that there is "incentive to learn how to manipulate the human," because predicting the human (on a wide range of inputs) is a very similar task to manipulating the human.
Okay, now I'll try to answer the question. I don't understand the question. I assume you are talking about incentive to manipulate in the simple examples with permutations etc in the experiments. I think there is no ability to manipulate those processes, and thus no gradient signal towards manipulation of the automated process. I still feel like there is some weird counterfactual incentive to manipulate the process, but I don't know how to say what that means, and I agree that it does not affect what actually happens in the system.
I agree that changing to a human will not change anything (except via also adding the change where the system is told (or can deduce) that it is interacting with the human, and thus ignores the gradient signal, to do some treacherous turn). Anyway, in these worlds, we likely already lost, and I am not focusing on them. I think the short answer to your question is in practice no, there is no difference, and there isn't even incentive to predict humans in strong generality, much less manipulate them, but that is because the examples are simple and not trying to have common information with how humans work.
I think that there are two paths to go down of crux opportunities for me here, and I'm sure we could find more: 1) being convinced that there is not an incentive to predict humans in generality (predicting humans only when they are very strictly following a non-humanlike algorithm doesn't count as predicting humans in generality), or 2) being convinced that this incentive to predict the humans is sufficiently far from incentive to manipulate.