I think the RLHF solves 80% of the problems of outer alignment, and I expect it to be part of the solution.
But :
- RLHF doesn't fully solve the difficult problems, which are beyond human supervision, i.e. the problems where even humans don't know what is the right way to do
- RLHF does not solve the problem of goodharting: For example there is the example of the hand which wriggles in front of the ball, without catching the ball and which fools the humans. (Imho I find this counter-example very weak, and I wonder how the human evaluators could miss this problem: it's very clear in the gif that the hand does not grab the ball).
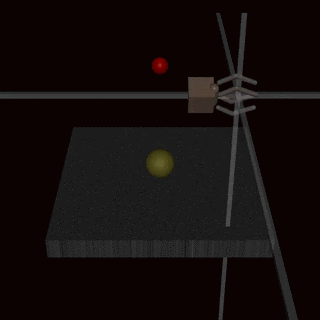
I have a presentation on RLHF tomorrow, and I can't understand why the community is so divided on this method.
The complex value paper is the obvious one, which as the name suggests talks about the complexity of value as one of the primary drivers of the outer alignment problem:
Eliezer isn't talking strictly about a reinforcement learning setup (but more a classification setup), but I think it comes out to the right thing. Hibbard was suggesting that you learn human values by basically doing reinforcement learning by classifying smiling humans (an approach that strikes as approximately as robust as RLHF), with Eliezer responding about how in the limit this really doesn't give you the thing you want.
In Robby's followup-post "The Genie knows but doesn't care", Eliezer says in the top comment: